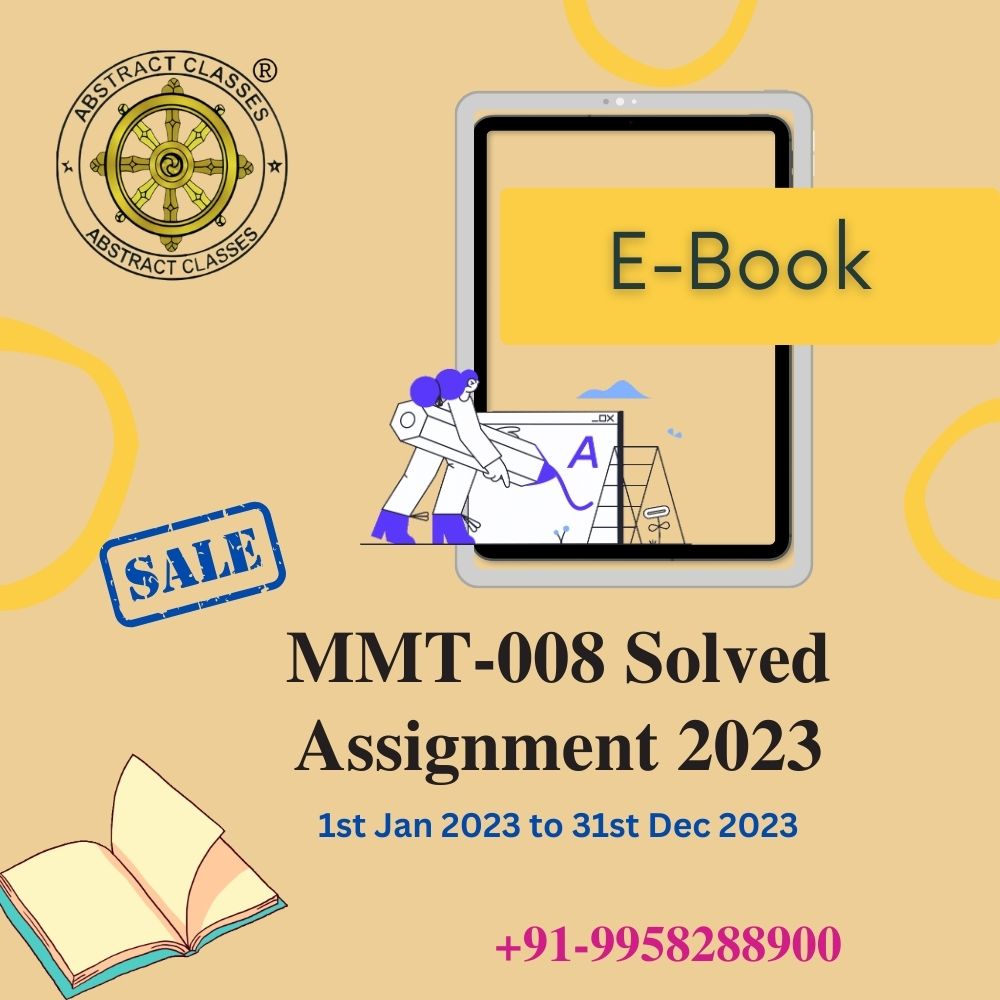
IGNOU MMT-008 Solved Assignment 2023 | M.Sc. MACS
₹365.00
Access via our Android App Only
Please read the following points before ordering :
Share with your Friends
IGNOU MMT-008 Assignment Question Paper 2023
- State whether the following statements are True or False. Justify your answer with a short proof or a counter example:
-
a) Let
(X,Y) (X, Y)
|
|||||
2 | 3 | 4 | 5 | ||
|
0 | 0 | 0.03 | 0 | 0 |
1 | 0.34 | 0.30 | 0.16 | 0 | |
2 | 0 | 0 | 0.03 | 0.14 |
-
a) Let
X∼N_(3)(mu,Sigma) \mathrm{X} \sim \mathrm{N}_{3}(\mu, \Sigma) mu=[5,3,4]^(‘) \mu=[5,3,4]^{\prime}
- a) Consider a Markov chain with transition probability matrix:
- a) A service station has 5 mechanics each of whom can service a scooter in 2 hours on the average. The scooters are registered at a single counter and then sent for servicing to different mechanics. Scooters arrive at a service station at an average rate of 2 scooters per hour. Assuming that the scooter arrivals are Poisson and service times are exponentially distributed, determine:
-
a) Let the random vector
X^(‘)=(X_(1),X_(2),X_(3)) X^{\prime}=\left(X_{1}, X_{2}, X_{3}\right) [-2,3,4] [-2,3,4] =([1,1,1],[1,2,3],[1,3,9]) =\left(\begin{array}{lll}1 & 1 & 1 \\ 1 & 2 & 3 \\ 1 & 3 & 9\end{array}\right) Y=b_(0)+b_(1)X+b_(2)X_(2) Y=b_{0}+b_{1} X+b_{2} X_{2} X_(3) \mathrm{X}_{3} [X_(1),X_(2)] \left[\mathrm{X}_{1}, \mathrm{X}_{2}\right]
-
a) If the random vector
Z \mathrm{Z} N_(4)(mu,Sigma) \mathrm{N}_{4}(\mu, \Sigma)
ii)
-
a) Consider the Markov chain with three states,
S={1,2,3} S=\{1,2,3\}
-
a) Let
X=[[X_(1)],[X_(2)]] X=\left[\begin{array}{l}X_{1} \\ X_{2}\end{array}\right] mu=[[0],[1]] \mu=\left[\begin{array}{l}0 \\ 1\end{array}\right] [[1,-1],[-1,2]] \left[\begin{array}{cc}1 & -1 \\ -1 & 2\end{array}\right] Y=AX+b \mathrm{Y}=\mathrm{AX}+\mathrm{b}
ii) Compute
iii) Find the covariance matrix of
iv) Find
A: first coin toss results in a head.
B: second coin toss results in a head.
C: coin 1 (regular) has been selected.
-
a) Consider three random variables
X_(1),X_(2),X_(3) X_{1}, X_{2}, X_{3}
MMT-008 Sample Solution 2023
Question:-01
-
State whether the following statements are True or False. Justify your answer with a short proof or a counter example:
a) IfP \mathrm{P} lim_(nrarr oo)P^(n) \lim _{\mathrm{n} \rightarrow \infty} \mathrm{P}^{\mathrm{n}}
Counterexample:
Conditions for the Statement to be True:
Page Break
Counterexample:
Additional Notes:
-
The diagonal elements of a variance-covariance matrix, which represent variances, are always non-negative because variance cannot be negative.
-
Off-diagonal elements, which represent covariances, can be negative, zero, or positive, depending on the relationship between the variables involved.
Page Break
Justification:
-
Mean : The mean of
(X_(1)+X_(2)+X_(3))/(3) \frac{X_1+X_2+X_3}{3} (mu+mu+mu)/(3)=mu \frac{\mu + \mu + \mu}{3} = \mu -
Covariance Matrix : The covariance matrix of
X_(1)+X_(2)+X_(3) X_1+X_2+X_3 Sigma+Sigma+Sigma=3Sigma \Sigma + \Sigma + \Sigma = 3\Sigma X_(1),X_(2),X_(3) X_1, X_2, X_3 (X_(1)+X_(2)+X_(3))/(3) \frac{X_1+X_2+X_3}{3} (1)/(3^(2))(3Sigma)=(1)/(3)Sigma \frac{1}{3^2}(3\Sigma) = \frac{1}{3}\Sigma
Page Break
Justification:
-
Partial Correlation Coefficients : The partial correlation coefficient measures the strength and direction of the relationship between two variables while controlling for the effect of one or more other variables. Mathematically, it is defined in a way that ensures its value lies between -1 and 1, inclusive. Specifically, it is computed as the correlation between the residuals resulting from the linear regression of each variable against the control variables. Since residuals are uncorrelated with the predicted values, the partial correlation coefficient is constrained to be between -1 and 1.
-
Multiple Correlation Coefficients : The multiple correlation coefficient
R R R^(2) R^2 R^(2) R^2 R R R R
Page Break
Justification:
Frequently Asked Questions (FAQs)
You can access the Complete Solution through our app, which can be downloaded using this link:
Simply click “Install” to download and install the app, and then follow the instructions to purchase the required assignment solution. Currently, the app is only available for Android devices. We are working on making the app available for iOS in the future, but it is not currently available for iOS devices.
Yes, It is Complete Solution, a comprehensive solution to the assignments for IGNOU. Valid from January 1, 2023 to December 31, 2023.
Yes, the Complete Solution is aligned with the IGNOU requirements and has been solved accordingly.
Yes, the Complete Solution is guaranteed to be error-free.The solutions are thoroughly researched and verified by subject matter experts to ensure their accuracy.
As of now, you have access to the Complete Solution for a period of 6 months after the date of purchase, which is sufficient to complete the assignment. However, we can extend the access period upon request. You can access the solution anytime through our app.
The app provides complete solutions for all assignment questions. If you still need help, you can contact the support team for assistance at Whatsapp +91-9958288900
No, access to the educational materials is limited to one device only, where you have first logged in. Logging in on multiple devices is not allowed and may result in the revocation of access to the educational materials.
Payments can be made through various secure online payment methods available in the app.Your payment information is protected with industry-standard security measures to ensure its confidentiality and safety. You will receive a receipt for your payment through email or within the app, depending on your preference.
The instructions for formatting your assignments are detailed in the Assignment Booklet, which includes details on paper size, margins, precision, and submission requirements. It is important to strictly follow these instructions to facilitate evaluation and avoid delays.
Terms and Conditions
- The educational materials provided in the app are the sole property of the app owner and are protected by copyright laws.
- Reproduction, distribution, or sale of the educational materials without prior written consent from the app owner is strictly prohibited and may result in legal consequences.
- Any attempt to modify, alter, or use the educational materials for commercial purposes is strictly prohibited.
- The app owner reserves the right to revoke access to the educational materials at any time without notice for any violation of these terms and conditions.
- The app owner is not responsible for any damages or losses resulting from the use of the educational materials.
- The app owner reserves the right to modify these terms and conditions at any time without notice.
- By accessing and using the app, you agree to abide by these terms and conditions.
- Access to the educational materials is limited to one device only. Logging in to the app on multiple devices is not allowed and may result in the revocation of access to the educational materials.
Our educational materials are solely available on our website and application only. Users and students can report the dealing or selling of the copied version of our educational materials by any third party at our email address (abstract4math@gmail.com) or mobile no. (+91-9958288900).
In return, such users/students can expect free our educational materials/assignments and other benefits as a bonafide gesture which will be completely dependent upon our discretion.
Related products
-
IGNOU Assignment Solution
IGNOU MEG-12 Solved Assignment 2022-2023 | MEG | A Survey Course in 20th Century Canadian Literature
₹101.00 Go to the App -
IGNOU Assignment Solution
IGNOU MEG-11 Solved Assignment 2022-2023 | MEG | AMERICAN NOVEL
₹101.00 Go to the App -
IGNOU Assignment Solution
IGNOU MEG-08 Solved Assignment 2022-2023 | MEG | NEW LITERATURES IN ENGLISH
₹101.00 Go to the App -
IGNOU Assignment Solution
IGNOU MEG-05 Solved Assignment 2022-2023 | MEG | LITERARY CRITICISM & THEORY
₹101.00 Go to the App