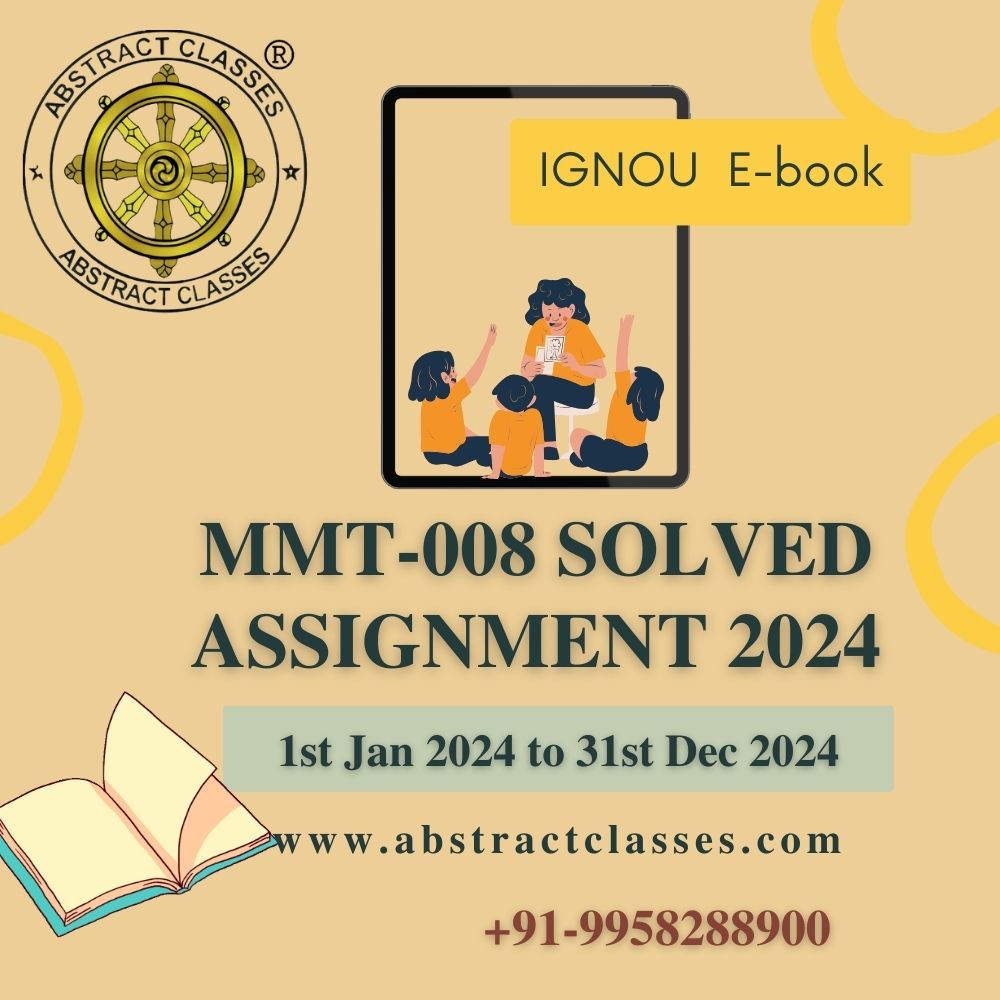
IGNOU MMT-008 Solved Assignment 2024 | M.Sc. MACS
₹365.00
Access via our Android App Only
Please read the following points before ordering :
Share with your Friends
IGNOU MMT-008 Assignment Question Paper 2024
mmt-008-assignment-question-paper-71fd4fc7-0370-49c3-9763-0667d1defc6c
- a) Consider the Markov chain having the following transition probability matrix.
ii) Classify the states of a Markov chain, i.e., persistent, transient, non-null and a periodic state. Also check the irreducibility of Markov chain.
iii) Find the closed sets.
iv) Find the probability of absorption to the closed classes. Also find the mean time up to absorption from transient state 3 to 4 .
b) Determine the parameters of the bivariate normal distribution:
2. a) Suppose that the probability of a dry day (State 0 ) following a rainy day (State 1 ) is
Given that
i) the probability that
ii) the stationary probabilities.
b) Let
i) Obtain the marginal distribution of
ii) Check the independence of
iii) Obtain the conditional distribution of
iv) Find
3. a) Suppose that customers arrive at a service counter in accordance with a Poisson process with the mean rate 2 per minute. Then obtain the probability that the interval between two successive arrivals is
i) more than 1 minute.
ii) 4 minutes or less.
iii) between 1 and 2 minutes.
b) Write two advantages and two disadvantages of conjoint analysis.
4. a) Find the differential equation of pure birth process with
b) Let
5. The body dimensions of a certain species have been recorded. The information of body length
|
|
||||
45 | 2.9 | ||||
48 | 2.4 | ||||
45 | 2.8 | ||||
48 | 2.9 | ||||
44 | 2.4 | ||||
45 | 2.3 | ||||
45 | 3.1 | ||||
42 | 1.7 | ||||
50 | 2.4 | ||||
52 | 3.7 |
[You may like to use the values,
6. The Tooth Care Hospital provides free dental service to the patients on every Saturday morning. There are 3 dentists on duty, who are equally qualified and experienced. It takes on an average 20 minutes for a patient to get treatment and the actual time taken is known to vary approximately exponentially around this average. The patients arrive according to the Poisson distribution with an average of 6 per hour. The officer of the hospital wants to investigate the following:
i) The expected number of patients in the queue.
ii) The average time that a patient spends at the clinic.
iii) The average percentage idle time for each of the dentists.
7. a) For the two-state Markov chain, whose transition probability matrix is
b) Let
i)
ii)
8. a) Let
b) For
- a) The joint density function of random variables
X,Y \mathrm{X}, \mathrm{Y} andZ \mathrm{Z} is given as
i) the constant
ii) the marginal distributions of
iii)
iv) the conditional expectation of
v) the correlation coefficient between
b) For the model
10. State which of the following statements are true and which are false. Give a short proof or a counter example in support of your answer.
a) For three independent events
c) If
d) In Hotelling
MMT-008 Sample Solution 2024
mmt-008-solved-assignment-2024-ss-8e24e610-06c9-4b43-84f6-a5bf6ef5ab5c
- a) Consider the Markov chain having the following transition probability matrix.
ii) Classify the states of a Markov chain, i.e., persistent, transient, non-null and a periodic state. Also check the irreducibility of Markov chain.
iii) Find the closed sets.
iv) Find the probability of absorption to the closed classes. Also find the mean time up to absorption from transient state 3 to 4 .
+--------+ +--------+ +--------+ +--------+ +--------+ +--------+
| 1 | ----> | 2 | ----> | 3 | ----> | 4 | ----> | 5 | ----> | 6 |
+--+-----+ +--+-----+ +--+-----+ +--+-----+ +--+-----+ +--+-----+
| \ | \ | \ | \ | \ | \
| 1/3 | ----> | 2/3 | ----> (0) | ----> (0) | ----> (0) | ----> (0)
| / | / | / | / | / | /
+--------+ +--+-----+ +--+-----+ +--+-----+ +--+-----+ +--+-----+
| | \ | \ | \ | \ | \
| \ | 1/4 | ----> | 1/4 | ----> | 1/4 | ----> | 1/4 |
| -----> | / | / | / | / | /
+--------+ +--+-----+ +--+-----+ +--+-----+ +--+-----+ +--+-----+
| | |
(1/6) | (1/6) | (1/6) | (1/6)
+--------+ +--------+
| 6 | ---- | 4 |
+--+-----+ +--+-----+
| |
| (3/4) |
| |
+--------+ +--------+
- Each circle represents a state (1 to 6).
- Arrows show the possible transitions between states.
- The number on each arrow represents the probability of that transition.
- States 3, 5, and 6 are absorbing states as they have no outgoing arrows.
- States 1, 2, and 4 are transient states as they can leave and enter again.
- Persistent States: These are states that, once entered, the process has a nonzero probability of staying in forever. In this chain, there are no persistent states.
- Transient States: These are states that, once left, the process has a zero probability of returning to. In this chain, states 1, 2, 3, and 4 are transient states.
- Null States: These are states that do not lead to any other state. In this chain, there are no null states.
- Periodic States: A state is periodic if the process can return to it only at multiples of some integer greater than 1. In this chain, there are no periodic states, as all states can potentially be returned to at any time.
- Irreducibility: A Markov chain is irreducible if it is possible to get from any state to any other state. This chain is not irreducible because there are states that cannot be reached from other states (e.g., there is no path from state 1 to state 6).
- {5, 6}
- From state 3, the probability of absorption to the closed class {5, 6} is 1, as there is a direct transition to state 5 with probability 1/6.
- From state 4, the probability of absorption to the closed class {5, 6} is also 1, as there is a direct transition to state 5 with probability 1/4.
- From state 3, the process moves to state 5 with probability 1/6 in one step, so the mean time to absorption is 1.
- From state 4, the process moves to state 5 with probability 1/4 in one step, so the mean time to absorption is also 1.
- Mean of
x x (mu _(x) \mu_x ): 7 - Mean of
y y (mu _(y) \mu_y ): -5 - Variance of
x x (sigma_(x)^(2) \sigma_x^2 ): The coefficient of(x-7)^(2) (x-7)^2 is(8)/(27) \frac{8}{27} , sosigma_(x)^(2)=(27)/(2xx(8)/(27))=(27)/(16) \sigma_x^2 = \frac{27}{2 \times \frac{8}{27}} = \frac{27}{16} , andsigma _(x)=sqrt((27)/(16))=(3sqrt3)/(4) \sigma_x = \sqrt{\frac{27}{16}} = \frac{3\sqrt{3}}{4} . - Variance of
y y (sigma_(y)^(2) \sigma_y^2 ): The coefficient of(y+5)^(2) (y+5)^2 is(32)/(27) \frac{32}{27} , sosigma_(y)^(2)=(27)/(2xx(32)/(27))=(27)/(64) \sigma_y^2 = \frac{27}{2 \times \frac{32}{27}} = \frac{27}{64} , andsigma _(y)=sqrt((27)/(64))=(3sqrt3)/(8) \sigma_y = \sqrt{\frac{27}{64}} = \frac{3\sqrt{3}}{8} . - Correlation coefficient (
rho \rho ): The coefficient of(x-7)(y+5) (x-7)(y+5) is-(16)/(27) -\frac{16}{27} , sorho=(-(16)/(27))/(2xx(3sqrt3)/(4)xx(3sqrt3)/(8))=-(1)/(2) \rho = \frac{-\frac{16}{27}}{2 \times \frac{3\sqrt{3}}{4} \times \frac{3\sqrt{3}}{8}} = -\frac{1}{2} .
mu _(x)=7 \mu_x = 7 mu _(y)=-5 \mu_y = -5 sigma _(x)=(3sqrt3)/(4) \sigma_x = \frac{3\sqrt{3}}{4} sigma _(y)=(3sqrt3)/(8) \sigma_y = \frac{3\sqrt{3}}{8} rho=-(1)/(2) \rho = -\frac{1}{2} k=(32)/(27 pisqrt3) k = \frac{32}{27\pi\sqrt{3}}
Frequently Asked Questions (FAQs)
You can access the Complete Solution through our app, which can be downloaded using this link:
Simply click “Install” to download and install the app, and then follow the instructions to purchase the required assignment solution. Currently, the app is only available for Android devices. We are working on making the app available for iOS in the future, but it is not currently available for iOS devices.
Yes, It is Complete Solution, a comprehensive solution to the assignments for IGNOU. Valid from January 1, 2023 to December 31, 2023.
Yes, the Complete Solution is aligned with the IGNOU requirements and has been solved accordingly.
Yes, the Complete Solution is guaranteed to be error-free.The solutions are thoroughly researched and verified by subject matter experts to ensure their accuracy.
As of now, you have access to the Complete Solution for a period of 6 months after the date of purchase, which is sufficient to complete the assignment. However, we can extend the access period upon request. You can access the solution anytime through our app.
The app provides complete solutions for all assignment questions. If you still need help, you can contact the support team for assistance at Whatsapp +91-9958288900
No, access to the educational materials is limited to one device only, where you have first logged in. Logging in on multiple devices is not allowed and may result in the revocation of access to the educational materials.
Payments can be made through various secure online payment methods available in the app.Your payment information is protected with industry-standard security measures to ensure its confidentiality and safety. You will receive a receipt for your payment through email or within the app, depending on your preference.
The instructions for formatting your assignments are detailed in the Assignment Booklet, which includes details on paper size, margins, precision, and submission requirements. It is important to strictly follow these instructions to facilitate evaluation and avoid delays.
Terms and Conditions
- The educational materials provided in the app are the sole property of the app owner and are protected by copyright laws.
- Reproduction, distribution, or sale of the educational materials without prior written consent from the app owner is strictly prohibited and may result in legal consequences.
- Any attempt to modify, alter, or use the educational materials for commercial purposes is strictly prohibited.
- The app owner reserves the right to revoke access to the educational materials at any time without notice for any violation of these terms and conditions.
- The app owner is not responsible for any damages or losses resulting from the use of the educational materials.
- The app owner reserves the right to modify these terms and conditions at any time without notice.
- By accessing and using the app, you agree to abide by these terms and conditions.
- Access to the educational materials is limited to one device only. Logging in to the app on multiple devices is not allowed and may result in the revocation of access to the educational materials.
Our educational materials are solely available on our website and application only. Users and students can report the dealing or selling of the copied version of our educational materials by any third party at our email address (abstract4math@gmail.com) or mobile no. (+91-9958288900).
In return, such users/students can expect free our educational materials/assignments and other benefits as a bonafide gesture which will be completely dependent upon our discretion.
Related products
-
IGNOU Assignment Solution
IGNOU MEG-16 Solved Assignment 2022-2023 | MEG | Indian Folk Literature
₹101.00 Go to the App -
IGNOU Assignment Solution
IGNOU MEG-10 Solved Assignment 2022-2023 | MEG | ENGLISH STUDIES IN INDIA
₹101.00 Go to the App -
IGNOU Assignment Solution
IGNOU MEG-02 Solved Assignment 2022-2023 | MEG | British Drama
₹101.00 Go to the App