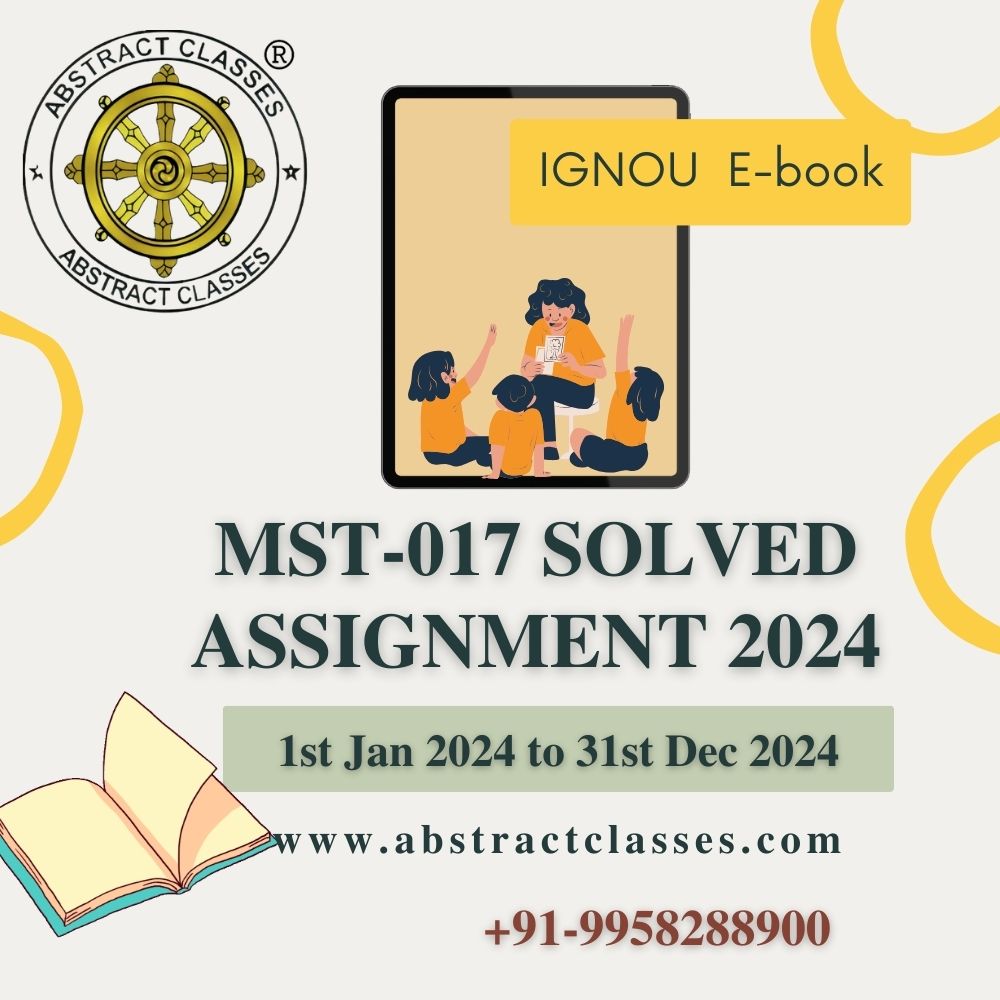
IGNOU MST-017 Solved Assignment 2024 | MSCAST | IGNOU
₹365.00
Access via our Android App Only
Please read the following points before ordering :
Share with your Friends
IGNOU MST-017 Assignment Question Paper 2024
mst-017-solved-assignment-2024-qp-8e24e610-06c9-4b43-84f6-a5bf6ef5ab5c
(ii) If the coefficient of determination is 0.833 , the number of observations and explanatory variables are 12 and 3 , respectively, then the Adjusted
(iii) For a simple regression model fitted on 15 observations, if we have
(iv) In a regression model
(v) The logit link function is
(b) Write a short note on the problem of multicollinearity and autocorrelation.
2(a) Explain the assumptions underlying multiple linear regression model.
(b) Suppose a researcher wants to evaluate the effect of cholesterol on the blood pressure. The following data on serum cholesterol (in
(iii) Test the significance of the fitted regression model.
- For the data given in Question 2(b), obtain the followings:
(i) Diagonal of the hat matrix and also check the leverage points if any.
(ii) Cook’s Distances, DFFITS and DFBETAS. Also verify the influence points if any.
Employee | Test 1 | Test 2 | Test 3 | Test 4 | Test 5 | IQ |
1 | 83 | 80 | 78 | 77 | 67 | 99 |
2 | 73 | 85 | 67 | 80 | 63 | 92 |
3 | 81 | 80 | 71 | 81 | 68 | 94 |
4 | 96 | 86 | 82 | 83 | 56 | 99 |
5 | 84 | 73 | 75 | 75 | 68 | 94 |
6 | 72 | 74 | 71 | 67 | 59 | 79 |
7 | 84 | 79 | 84 | 84 | 69 | 97 |
8 | 54 | 86 | 61 | 69 | 53 | 92 |
9 | 86 | 85 | 79 | 78 | 76 | 94 |
10 | 42 | 71 | 60 | 80 | 56 | 86 |
11 | 83 | 72 | 72 | 78 | 74 | 98 |
12 | 63 | 86 | 65 | 85 | 56 | 83 |
13 | 69 | 76 | 64 | 85 | 61 | 98 |
14 | 81 | 84 | 65 | 79 | 64 | 96 |
15 | 50 | 85 | 71 | 65 | 75 | 76 |
- The following data on diagnosis of coronary heart disease (where 0 indicating absence and 1 indicating presence), serum cholesterol (in
mg//dl \mathrm{mg} / \mathrm{dl} ), resting blood pressure (inmmHg \mathrm{mmHg} ) and weight (inkg \mathrm{kg} ) were obtained for 80 patients to explore the relationship of coronary heart disease with cholesterol and weight:
|
|
|
|
|
|||||||||||||
1 | 420 | 60 | 10 | 20 | |||||||||||||
2 | 450 | 68 | 15 | 30 | |||||||||||||
3 | 400 | 54 | 4 | 15 | |||||||||||||
4 | 510 | 74 | 2 | 10 | |||||||||||||
5 | 480 | 62 | 1 | 5 |
(ii) Test the significance of the fitted model using Hosmer-Lemeshow test at
MST-017 Sample Solution 2024
mst-017-solved-assignment-2024-ss-8e24e610-06c9-4b43-84f6-a5bf6ef5ab5c
(i) We define three indicator variables for an explanatory variable with three categories.
Statement Analysis
Why Only n-1 n-1 Indicator Variables?
D1=1 D1 = 1 if the category is A, andD1=0 D1 = 0 otherwise.D2=1 D2 = 1 if the category is B, andD2=0 D2 = 0 otherwise.
Conclusion
Coefficient of Determination (R^(2) R^2 )
Adjusted R^(2) R^2
n n is the number of observations,k k is the number of explanatory variables,R^(2) R^2 is the coefficient of determination.
R^(2)=0.833 R^2 = 0.833 ,n=12 n = 12 (number of observations),k=3 k = 3 (number of explanatory variables).
Calculation
Conclusion
Leverage Values
Analysis of the Statement
- A simple regression model (which means
p=1 p = 1 predictor plus the intercept). - Fitted on 15 observations (
n=15 n = 15 ). - A specific observation has
h_(ii)=0.37 h_{ii} = 0.37 .
Conclusion
Frequently Asked Questions (FAQs)
You can access the Complete Solution through our app, which can be downloaded using this link:
Simply click “Install” to download and install the app, and then follow the instructions to purchase the required assignment solution. Currently, the app is only available for Android devices. We are working on making the app available for iOS in the future, but it is not currently available for iOS devices.
Yes, It is Complete Solution, a comprehensive solution to the assignments for IGNOU.
Yes, the Complete Solution is aligned with the requirements and has been solved accordingly.
Yes, the Complete Solution is guaranteed to be error-free.The solutions are thoroughly researched and verified by subject matter experts to ensure their accuracy.
As of now, you have access to the Complete Solution for a period of 1 Year after the date of purchase, which is sufficient to complete the assignment. However, we can extend the access period upon request. You can access the solution anytime through our app.
The app provides complete solutions for all assignment questions. If you still need help, you can contact the support team for assistance at Whatsapp +91-9958288900
No, access to the educational materials is limited to one device only, where you have first logged in. Logging in on multiple devices is not allowed and may result in the revocation of access to the educational materials.
Payments can be made through various secure online payment methods available in the app.Your payment information is protected with industry-standard security measures to ensure its confidentiality and safety. You will receive a receipt for your payment through email or within the app, depending on your preference.
The instructions for formatting your assignments are detailed in the Assignment Booklet, which includes details on paper size, margins, precision, and submission requirements. It is important to strictly follow these instructions to facilitate evaluation and avoid delays.
Terms and Conditions
- The educational materials provided in the app are the sole property of the app owner and are protected by copyright laws.
- Reproduction, distribution, or sale of the educational materials without prior written consent from the app owner is strictly prohibited and may result in legal consequences.
- Any attempt to modify, alter, or use the educational materials for commercial purposes is strictly prohibited.
- The app owner reserves the right to revoke access to the educational materials at any time without notice for any violation of these terms and conditions.
- The app owner is not responsible for any damages or losses resulting from the use of the educational materials.
- The app owner reserves the right to modify these terms and conditions at any time without notice.
- By accessing and using the app, you agree to abide by these terms and conditions.
- Access to the educational materials is limited to one device only. Logging in to the app on multiple devices is not allowed and may result in the revocation of access to the educational materials.
Our educational materials are solely available on our website and application only. Users and students can report the dealing or selling of the copied version of our educational materials by any third party at our email address (abstract4math@gmail.com) or mobile no. (+91-9958288900).
In return, such users/students can expect free our educational materials/assignments and other benefits as a bonafide gesture which will be completely dependent upon our discretion.
Related products
-
IGNOU Assignment Solution
IGNOU MEG-18 Solved Assignment 2022-2023 | MEG | American Poetry
₹101.00 Go to the App -
IGNOU Assignment Solution
IGNOU MEG-12 Solved Assignment 2022-2023 | MEG | A Survey Course in 20th Century Canadian Literature
₹101.00 Go to the App -
IGNOU Assignment Solution
IGNOU MEG-08 Solved Assignment 2022-2023 | MEG | NEW LITERATURES IN ENGLISH
₹101.00 Go to the App